4 minute read
Here’s a thought-provoking fact at the top of IBM’s infographic on Addressing complexity for automotive software and systems engineers:
Today’s cars have roughly 30,000 parts and more than 100 million lines of code.
Now connect this kind of engineering maze to the recent MIT Technology Review Insights white paper, “Optimizing the engineering life cycle requires digital transformation,” produced in association with IBM:
Organizations are plugging into IoT technology and AI-based data analytics to understand how products are actually being used by customers, and to adjust product development accordingly, in a cycle of continuous improvement. AI is being deployed in a variety of ways, from expert systems that help junior engineers write better requirements to machine learning that analyzes large data sets in order to spot patterns and help optimize processes.
The summation? Automotive engineering processes that are already complex only promise to get more complicated. And as they do, IoT technology, AI, machine learning, and data analytics will continue to play an increasingly central role. To seize the digital transformation in auto engineering, as IBM’s infographic also says, you need the right mix of tools and practices — Cloud-based, Analytics, Traceability, Modeling, Security, and Agile.
Here’s the comprehensive take on each one.
Cloud-based
Especially for requirements, engineering teams can use cloud-based AI systems to analyze requirements in real time as they’re being written. Teams can also use AI solutions to encode standards and best practices in order to automate lower-level processes, which frees up engineers to concentrate on actual design activities. For global engineering operations using IBM’s recently upgraded Enterprise Lifecycle Management (ELM) solution, cloud-based AI requirements management (DOORS/DOORS Next/RQA) and other engineering functions for design, testing and workflow management can be extended across geographies and time zones as well as disciplines and supply chains. (This additionally plays into the Agile component). Ultimately, when the finished product is a smart car, high-speed cloud connectivity is the backbone for the vehicle’s real-time diagnostics via IoT technology, embedded sensors and AI-driven data analytics.
Analytics
With so much data coming at software and systems engineers in the auto industry, machine learning has become their saving grace. Moreover, with best case uses for data analytics trending from a predictive model to a prescriptive one, machine learning is taking on added importance. IBM defines prescriptive analytics as Optimization to achieve the best outcome, plus Stochastic Optimization to achieve the best outcome by accounting for uncertainty in existing data. In effect, rather than predicting an outcome, prescriptive analytics tweak specific variables to achieve the best possible result, and then prescribe the course of action accordingly to make better decisions.
For automotive engineering design, IBM Watson is one machine learning solution that makes prescriptive analytics viable. So do other tools, according to Gartner’s definition of prescriptive analytics. Graph analysis, simulation, complex event processing, recommendation engines, heuristics, and neural networks also provide combinations of various machine learning and AI algorithms to process large amounts of complex data. Used in a prescriptive approach, these tools could help teams improve requirements accuracy, design outcomes, test methods and other engineering processes.
Traceability
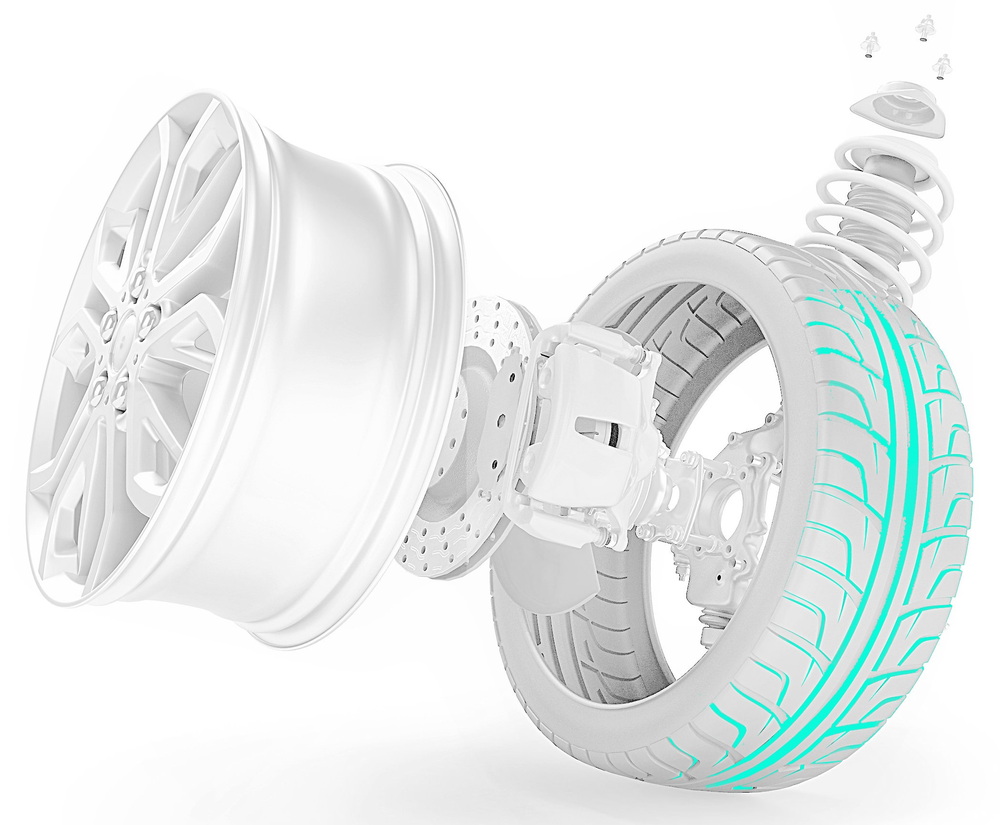
To gain total visibility of changes in product engineering requirements as they happen, IBM recommends linking individual artifacts to test cases throughout the development lifecycle. Creating and maintaining relationships across the dev lifecycle is additionally useful, meaning the relationships between requirements, work items, architecture, design and test plans. This is the tenant of IBM’s newly upgraded Enterprise Lifecycle Management (ELM) solution. ELM integrates technologies including the Watson AI-powered Requirements Quality Assistant (RQA) to improve the quality of complex requirements and advance automotive compliance to fast-track ASPICE compliance.
Modeling
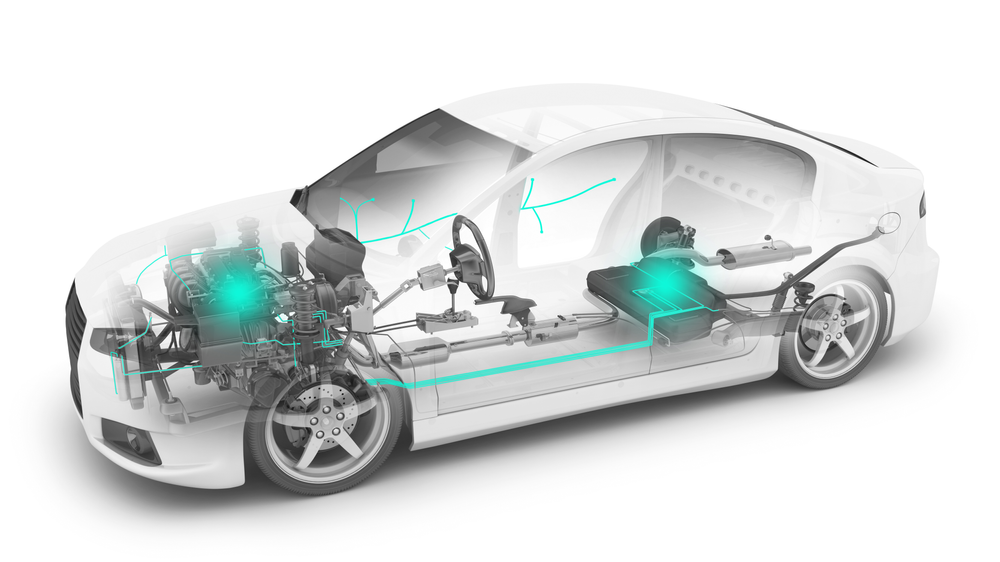
Digital modeling systems enable engineering teams to perform millions of simulations and test runs, and do so in a much shorter timeframe by combining simulations and running multiple iterations. Teams can also easily extend AI-based processes to modeling, as well as to quality assurance and testing, and integrate them into one system. With such a system and AI to analyze engineering processes as they’re being implemented, team members can scrutinize the maturity of requirements, spot trends, and identify programs that might be at risk.
A further benefit is being able to link engineering models to the manufacturing process. Collectively, a modeling system featuring AI can help engineering groups automate test procedures, analyze whether a part passed or failed a test, make needed corrections, and retest the corrected design. This kind of system could even autogenerate all lines of code required by the manufacturing software systems.
Security
Security can be viewed in two ways. For an engineering environment, a cloud infrastructure can provide a higher level of security via the cloud’s inherent security mechanisms. IBM ELM Managed Services by ClearObject, for example, provide the security of a single-tenant virtual private cloud for deployment and to monitor cloud and user activity, with the added protection of a VPN tunnel. For the security, and safety, of vehicles — particularly autonomous vehicles that incorporate IoT sensors and AI-based data analytics — such vehicles are basically rolling connected devices and prime targets for cyberattacks. Security therefore is paramount.
To address the issue, the auto industry is set to adopt the new ISO/SAE 21434 cyber security standard in mid-2020. The standard is intended to create industry-wide agreement on cyber security issues, and ensure that the global supply chain has processes in place that support a security by design approach. ISO/SAE 21434, which applies to a vehicle’s components, connections and software, considers the entire development process and life cycle of a vehicle. Under this new standard, manufacturers will need to meet a security-aware requirements analysis and product specification to build secure vehicle models.
Agile
Agile management should streamline product engineering processes and enable global collaboration along with a single source of truth, according to IBM. This includes showing teams the “value of their efforts” in real time. Agile enables collaboration internally with all stakeholders and engineering team members and externally with manufacturers, designers, regulators, suppliers, and so on. With a central requirements repository (single source of truth), agile also keeps large, diverse teams synchronized throughout the engineering and development process. This speeds consolidation, monitoring and analysis and ensures consistent and accurate information.
To manage requirements in an agile environment, using AI and data analytics helps reduce ambiguity and improve requirements accuracy (and ultimately product quality); capture, trace, analyze and manage requirements more accurately; and enhance operational data/customer insight to improve engineering decisions.
When engineering operations are global, consider cloud-based managed services to work more effectively across disciplines, time zones, and supply chains. This condenses requirements review cycles and improves collaboration even when different time-zones are involved. Also, along with a more secure environment for collaboration and data sharing, cloud-based managed services help ensure engineering environment reliability and uptime, typically with follow-the-sun support. Global engineering operations can even scale as needed, quickly, anywhere in the world.
IBM ELM Managed Services by ClearObject
As both an IBM Gold Business Partner and the sole managed service provider for IBM SaaS globally, ClearObject brings knowledge and responsiveness together in the form of the IBM development process and IBM Support. Also as a single trusted source, we keep your environment for software engineering and product development healthy and performing at peak efficiency.
About the Author
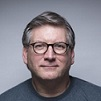
Tom Bailey is the Content Marketing Manager at ClearObject and has been writing about innovative technologies in the software and engineering industries for nearly 25 years.
About ClearObject
ClearObject is a digital transformation leader in Internet of Things (IoT) Engineering and Analytics. As IBM Watson IoT and Google Cloud Business Partners, we deliver global embedded software development environments for our customers, and design and deliver unique data analytics digital products that help them recognize the value of their data. Our objective is clear: help the world’s best companies build intelligence into their products and gain intelligence from them. The future is clear. Do you see it?
(Top image: IBM)
#iottechnology #machinelearning #dataanalytics