4 minute read
IoT keeps branching out. As more and more data, connected IoT devices and solutions for AI and machine learning have permeated the healthcare industry, the Internet of Medical Things (IoMT) has formally taken its place on the global technology map.
By definition (credit Andrew Keller), IoMT encompasses “devices that can collect and exchange data — either with users or other devices — via the internet. They are then used to allow doctors to be more aware of a patient’s condition on real-time basis.”
Primarily, IoMT technology improves how patients’ conditions are monitored and how doctors, nurses and other healthcare professionals are notified in the event of an emergency. Among IoT devices and IoT applications in the current IoMT realm are wearables (think diabetes monitors and personal fitness trackers), drug tracking systems, records management systems, medical supply chain solutions, remote patient monitoring devices and the like. IoMT is also increasingly making its way into smart hospitals and healthcare facilities.
The plusses of IoMT are that connected IoT devices and sensors can constantly collect and analyze health and fitness data in real time, monitor health changes in patients, and increase the accuracy of a patient’s diagnoses. It can connect entire networks of medical devices worldwide. It can locate healthcare-related assets and streamline patient care and medical research. In conjunction with IoT devices and IoT applications, AI and machine learning can help make processes more efficient for medical organizations and workers who support thousands of patients and manage sizeable amounts of data.
Yet as it is with any technology, IoMT is still advancing and continues to introduce some intriguing solutions by way of IoT for healthcare. Here are five recent developments that signal IoMT as being just as endless in its possibilities as IoT itself.
RYC™ from KeyAsic
KeyAsic was one of the featured innovators at CES 2020, and they’re doing some extremely valuable things for the medical industry using IoT and AI. For patients and their data in particular, the company just brought to market a device called Ramon Y Cajal, or simply RYC™. The solution consists of two components.
The first component is a patented device that can integrate a patient’s full Electronic Medical Records (EMR) library, including the ability to receive the person’s EMR from any clinic or hospital. An EMR is stored on this first device temporarily, and then uploaded to the second component of the RYC solution, the imedic™ Cloud. The imedic Cloud gives the patient full control of and access to their medical records, along with the ability to share the entire EMR with their physician through the imedic™ DoctorApp.
Other similar systems for medical records management and IoT in healthcare are Electronic Healthcare Records (EHR) systems. EHR platforms store information about patients and can provide real-time patient info instantly and securely to authorized users, such as doctors, nurses, and so on. These users can also share the patient’s data among various specialists to achieve better treatment results. The difference between EHR systems and the RYC EMR system from KeyAsic is that RYC gives patients themselves more control over, and much easier access to, their own medical records.
Ambient clinical intelligence
This concept comes from a Harvard Business Review article, distributed in part by healthcare device maker, Medtronic. The article, titled How AI in the Exam Room Could Reduce Physician Burnout, introduces ambient clinical intelligence (ACI) as a way to reduce voluminous paperwork and give doctors and nurses more time with patients. As the article’s authors write, “Accordingly, healthcare IT development should begin with a deep understanding of how clinicians need and want to work, then implement AI (and machine learning) capabilities with the explicit goal of adapting to and supporting how clinicians deliver care. ACI is one promising approach.”
Summarized from the article: ACI is less a device than a set of capabilities. The best way to picture ACI is to think of an exam room with a flat screen display to show a patient’s information. Small microphones capture a patient-doctor (or nurse) interaction accurately and in real time, regardless of the speakers’ movements or positions within the exam room. Since computing and data entry takes place in backend and cloud-based systems, no computer is required in the room.
Specifically, ACI builds on the same speech recognition technology that doctors have used for years. To authenticate clinical users, ACI uses voice biometrics to identify individuals by voice, along with other voice-based technologies to distinguish between the clinician, the patient, and anyone else in the exam room.
ACI further integrates conversational AI, machine learning, speech synthesis, natural language understanding, and cloud computing to provide clinical intelligence for diagnostic guidance. Such guidance can include pinpointing potentially overlooked diagnoses based on a patient’s health history and symptoms, as well as potential drug interactions and recommended alternative medications.
The most notable benefits of an ACI system are that it “responds” in real-time, say when a doctor or nurse asks to view patient histories or test results, orders tests, prescribes medications, and schedules follow-up appointments. Once a patient’s visit is completed, the system immediately and automatically creates a summary of the visit for the patient, updates the patient’s record, and enters appropriate billing codes for a physician’s office staff to review, edit, and submit in the electronic health record (EHR). Because patient privacy is integral to ACI for HIPAA compliance, all patient data collected in ACI must be closely guarded and stored with the patient’s consent. Data can then be repurposed only in anonymized form for clinical purposes, which must be clearly defined.
Data overload and doctors as data scientists
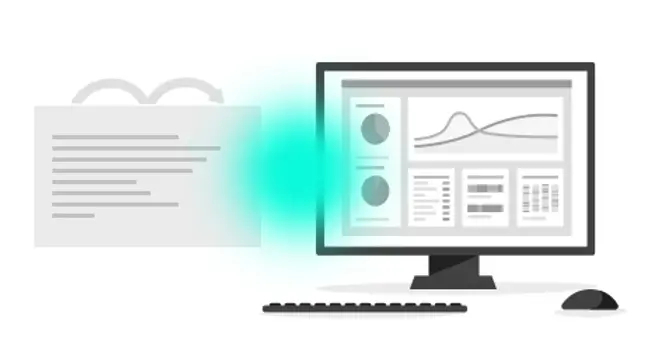
For IoMT, IoT devices and IoT applications collect and process literally ginormous volumes of information. The result for doctors in particular is data overload and questionable accuracy in the data they ultimately use. The bigger impact is how these reams of data affect decision-making during a patient’s treatment.
As one doctor framed his opinion on diagnostics based on the automated analysis of patient data, “The problem is liability. If I’m legally liable for my patient outcomes, I am not going to cede my professional autonomy to data science.” It’s a strong and valid argument shared by doctors throughout the healthcare community. But the issue of doctors as data scientists also is one that could conceivably introduce whole new methods of patient interaction, driven by an equally new class of medical workers known as personalized preventative health coaches.
Dr. Dimiter V. Dimitrov, MD, PhD, outlines this idea in a Healthcare Research Information article, suggesting that big data and IoT in healthcare will be the primary drivers for personalized preventative health coaches. Such coaches of course would need to possess the ability to interpret health and well-being data, which is where a data scientist role comes in. At the same time, though, health coaches would still have to be a “doctor” in helping patients improve their physical and mental health and their lifestyle overall. As the global population ages, Dr. Dimitrov says, personal preventative health coaches will play increasingly important roles.
There are technology challenges, however. Dr. Dimitrov cites the adoption of IoT in healthcare and ensuring the levels of connectivity required to gather, manage and analyze data as being a central hurdle. He further points to the need for easy device management, APIs that could streamline data aggregation into cloud services from various sources and platforms; actionable analytics; and reduced risk based on the ability to “act on notifications and isolate incidents generated anywhere in a healthcare environment from a single console.”
Dr. Dimitrov suggests a proactive approach to IoT in healthcare. “The traditional method of recording a patient’s details, i.e., a pad of paper hanging on the patient’s bed, is not going to work anymore since such records are only accessible to a limited few, and can be lost or scrambled…Health data information will be available in just a tap when information is recorded electronically, once security and privacy issues are met.”
To which we say, hello to IoMT, AI, machine learning, and innovations such as RYC™ from KeyAsic and ambient clinical intelligence.
Amazon Care
What does Amazon not do? Now the company is piloting a new employee program called Amazon Care, which equips workers with IoT devices to use when they’re sick.
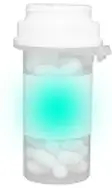
Essentially, Amazon Care is an extension of a traditional health insurance plan, in this case Amazon’s plan. The IoT-based benefit of Amazon Care is a smartphone app that connects the plan’s participants to the care they need from medical professionals, online or in-person. Participants can use Amazon Care for urgent care, scheduled doctor visits, issues related to preventive medicine like vaccinations and lab work, and so on. If a participant’s diagnosis requires treatment with prescription medicine, an Amazon “Care Courier” can bring prescribed meds to the patient’s home or office, often within two hours. Or, the prescription can be dispatched to a local pharmacy where the person can pick up the medication later.
Amazon Care works like this. When a plan participant (user) reports they have a health concern, the Amazon Care app screen presents the options of talking to a healthcare provider via text chat or video stream. In turn, the medical professional being contacted uses one of those methods to communicate with the patient to learn more about what they need. If it’s determined the patient/user rquires a more thorough face-to-face evaluation, a health professional is dispatched to the user’s location. The Amazon Care app additionally provides a real-time map tracking where the health professional is, including their estimated arrival time.
In the name of virtual doctors and convenient healthcare, Amazon Care is one of many new IoT applications in the IoMT movement allowing doctors and patients to stay in touch outside of a medical facility via monitoring and messaging components. For Amazon Care specifically, a summary component of the app that contains all details of a person’s diagnosis, notes from the doctor, and the patient/user’s recommended treatment plan. Beyond the Amazon Care app, users can view their summary info in a PDF.
Connected device maintenance
One thing many patients don’t think about is the equipment hospitals and labs and doctor’s offices use for diagnostics. Keeping this kind of equipment healthy and performing at peak efficiency is yet another case for IoT in healthcare, connected IoT devices, and the continued emergence if IoMT — and ClearObject is glad to say we’re doing our part.
One major device maker we work with manufacturers high-volume diagnostics testing systems used worldwide. For device maintenance, we’ve helped the company develop a way to compile and analyze sensor-based historical service information from these systems to improve on-site repair outcomes and reduce system downtime. The cloud-based predictive modeling solution for this client effectively recognizes keywords from service logs entered into a CRM system, and then predicts the root cause of failure in specific diagnostic systems. The model also recommends the precise corrective action for a service technician to take once they’re on site.
Another client we work with is known worldwide for providing cryofreezer data storage and safety through its CryoIntelligence network. Along with monitoring and recording critical data for biotech research institutions, hospitals, and pharma companies, this company provides services for cryogenic applications, data collection, computer interfacing sensing technologies, and the cloud storage of data.
We’ve helped this client enhance their CryoIntelligence application and its mechanisms for ingesting data from the connected devices their customers use to monitor cryofreezer systems. A key objective for the application upgrade was to be able to generate visible patterns in cryofreezer data. Such patterns, which require as much data as possible, are necessary for higher-level research in medical labs and for on-site system troubleshooting and repairs by service technicians. Machine learning isn’t part of this solution equation just yet, but managing and analyzing vast amounts of data to detect visible patterns in that data is just what machine learning is made for.
Is all of this kind of stuff where IoT in healthcare and IoMT is headed? As we said, the possibilities for IoMT are becoming just as endless as they are for IoT itself.
About the Author
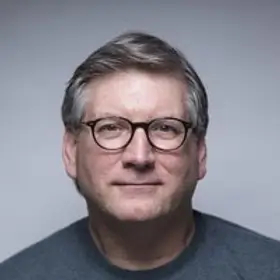
Tom Bailey is the Content Marketing Manager at ClearObject and has been writing about innovative technologies in the software and engineering industries for nearly 25 years.
About ClearObject
ClearObject is a digital transformation leader in Internet of Things (IoT) Engineering and Analytics. As IBM Watson IoT and Google Cloud Business Partners, we deliver global embedded software development environments for our customers, and design and deliver unique data analytics digital products that help them recognize the value of their data. Our objective is clear: help the world’s best companies build intelligence into their products and gain intelligence from them. The future is clear. Do you see it?
#iotinhealthcare #iomt #iotdevices #iotapplications #machinelearning