Prescriptive analytics are set to transform business intelligence and the way business leaders make decisions. And as more data analytics tools become available for prescriptive methods, don’t be surprised to see the model become a holy grail in industries of all kinds.
For what ClearObject views as the Year of IoT and Machine Learning in 2020, prescriptive analytics is one of the data-driven directions we see as steering the next phase of the digital transformation. Not that the model is some new cutting-edge business approach. It isn’t.
The term itself dates back to a 2010 article in Analytics Magazine written by a team from IBM; prescriptive analytics have been in use ever since. Yet for various reasons, it’s taken the method until now to hit its stride.
The turning point, at least according to the IBM authors, has been the amped-up data analytics power from technologies like IoT and machine learning for “comprehensive prescriptions.” We agree with the IBM team — but also add this: As big data becomes ginormous data, there will be much more data to analyze in prescriptive, forward-looking fashion.
This means more data that businesses can use to identify future trends and target where those trends will lead to. For business leaders, it means the ability to formulate more progressive strategies that pinpoint when and how to capitalize on forthcoming opportunities.
The peak of business analytics and business intelligence
By providing foresight and not just insight, prescriptive analytics is the peak of business analytics and business intelligence. A cheat sheet from TechRepublic puts prescriptive analytics and business analytics in perspective. As a multi-stage process, the cheat sheet says, business analytics involves analyzing data to reach conclusions of a particular type, allowing the business to take a collective strategic approach to drive organizational action.
Business analytics typically consist of these three models:
Descriptive analytics, which let an organization assess its current circumstances by analyzing records of past events — customer comments, product and services reviews, NPS scores, website traffic, Sales & Marketing numbers and so on. Essentially, descriptive analytics reflect the state of the business up to the present time.
Predictive analytics, which predict what will happen based on the business’s current circumstances, i.e., what will happen if current organizational practices stay as they are. This model uses the same type of data as descriptive analytics, sometimes including descriptive outcomes themselves, and many businesses now make machine learning and various forms of predictive modeling integral to the process.
Prescriptive analytics, which, instead of predicting an outcome, tweak specific variables to achieve the best possible result and then prescribe the course of action accordingly. IBM further defines prescriptive analytics as consisting of optimization, or how to achieve the best outcome, and stochastic optimization, or how to achieve the best outcome by accounting for uncertainty in existing data in order to make better decisions.
There’s also this note from TechRepublic’s prescriptive analytics cheat sheet. Whereas businesses can apply one or all three forms of analytics in the business analytics approach, they must do so in sequence. The reason is two-fold. First, predicting the future requires knowing what’s already happened, and second, changing course requires knowing what’s likely to happen without a course correction.
Should they choose to, decision-makers can discontinue the business analytics process once they get an accurate picture of their organization’s present state and what led up to it. This amounts to a limited descriptive analysis, however, which would be short-sighted and leave the business with a future of uncertainty and guesswork.
So bottom line, while descriptive analytics and predictive analytics provide insight, prescriptive analytics offer foresight that’s far more valuable for business intelligence that looks ahead.
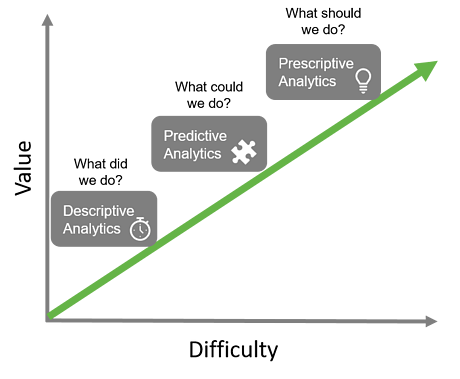
Finally becoming the data analytics benchmark
As we said, prescriptive analytics is on its way to becoming a holy grail thanks to greater volumes of available data and things like IoT, machine learning, and expanding suites of prescriptive analytics tools. These technologies all feed into the core of building a prescriptive model and its idea of optimizing factors of every kind. Workforce availability, production costs, scheduling, supply chain management, the potential failure of machinery…
For the data behind such factors, much of it can come from an IoT platform, connected devices and IoT edge devices. Connected IoT sensors in production equipment and transportation fleets also provide data for potential equipment failure, scheduled maintenance and so forth. Add the growing use of Data as a Service (DaaS) and the projection that every person in the world will create 1.7MB of data per second in 2020, and it only makes sense to use all this data in a prescriptive analytics environment to make more knowledgeable business decisions.
Machine learning: the driving force for prescriptive analytics
The problem with growing volumes of data is, there’s already more data available than humans can make sense of, especially in trying to identify analytical patterns for decision making. Which is why machine learning is being more broadly adopted both for prescriptive analytics and predictive analytics. (Resources like Google Cloud Machine Learning and IBM Watson have also made integrating machine learning much easier for prescriptive analytics.)
Early on, machine learning was viewed as a technology to automate tasks and replace human work. However, with the focus now on ML’s ability to augment human work, it makes processes like prescriptive analytics more efficient and employees more productive. And considering that more data makes for better prescriptive outcomes, this is one reason machine learning and AI are driving forces in the growth of prescriptive analytics — if not the driving forces.
Along with machine learning, Gartner’s definition of prescriptive analytics includes other tools that can lend to making the approach successful: Graph analysis, simulation, complex event processing, recommendation engines, heuristics, and neural networks, which provide combinations of various machine learning algorithms designed to process complex data.
Some real-world use cases for prescriptive analytics
Courtesy of TechRepublic’s prescriptive analytics cheat sheet …
Transportation and shipping companies (see IBM’s transportation case study and logistics study) use prescriptive analytics to:
- Improve driver retention, in turn reducing training costs for new hires
- Eliminate unnecessary driving, flight, and sea transportation miles
- Improve routes and eliminate wait times to load/unload
- Optimize distribution networks
- Eliminate nearly all warehouse packing errors; companies in the case study were 99.5% error free
The oil and gas industry uses prescriptive analysis extensively to:
- Improve drilling completion rates; machine learning models are trained to recognize the most beneficial places to set up field operations
- Determine the best possible locations in a specific oil field to drill first
- Optimize equipment configuration, which minimizes breakage, maintenance and downtime
- improve operational safety and eliminate potential environmental disasters
- Establish the best possible pricing by predicting the rise and fall of fuel markets
Financial services and banking, both described in IBM case studies, also use prescriptive analysis to:
- Decrease transaction processing time
- Reduce transaction costs
- increase the total amount of possible transactions processed in a set time period
- Create better portfolios and enhance existing ones for financial investment
- Optimize financial decisions: when to invest, how much to invest, etc.
- Reduce investment risk; per the IBM case study, prescriptive analysis reduced risk by nearly one third while maintaining similar yields
Contact us
ClearObject knows all about machine learning solutions for prescriptive analytics as both an IBM Gold Business Partner and certified Google Cloud Partner. In addition to IoT Engineering, Analytics and Connected Product Development, we’re experts at developing and implementing targeted data analytics strategies to get the most value from your data — including deploying and managing serverless cloud solutions for AI tools and end-to-end machine learning models.