Generative Artificial Intelligence (GenAI) has revolutionized various industries, enabling machines to create content that resembles human-generated data. From creating art and generating realistic images to crafting engaging stories, generative AI has pushed the boundaries of technological innovation. However, the remarkable advancements in this field have raised significant concerns regarding security and data privacy. In this blog post, we will explore these concerns and examine how companies are addressing them. Additionally, we will compare the approaches taken by Google, OpenAI, and Meta AI in handling security and privacy.
Security Concerns in Generative AI
Data Exposure: Generative AI models often require large datasets for training. The availability and utilization of extensive personal or sensitive data for training these models pose significant risks. If not handled properly, the training data can expose personal information, leading to potential privacy breaches or unauthorized access.
Adversarial Attacks: GenAI models can be vulnerable to adversarial attacks, where malicious actors manipulate the input data to trick the model into generating inappropriate or harmful content. These attacks can exploit model weaknesses, leading to the generation of biased, offensive, or even dangerous outputs.
Intellectual Property (IP) Theft: Generative AI models can inadvertently generate content that infringes on intellectual property rights. For instance, an AI-generated artwork might unintentionally resemble an existing copyrighted piece, potentially leading to legal consequences and disputes.
Data Privacy Concerns in Generative AI
Informed Consent: The use of personal data for training GenAI models necessitates informed consent from individuals whose data is being utilized. Obtaining explicit consent ensures that individuals understand the purpose and potential risks associated with their data usage.
Data Retention: Proper data retention policies need to be implemented to safeguard privacy. Generative AI models should not retain personal data longer than necessary, reducing the risk of unauthorized access and potential misuse.
Anonymization and De-identification: Personal information within the training datasets should be effectively anonymized or de-identified to protect the privacy of individuals. This process ensures that the generated outputs cannot be traced back to specific individuals, maintaining confidentiality.
Enterprise Security Measures for Generative AI
Generative AI has gained significant traction in the enterprise sector, offering transformative opportunities for businesses across various domains. However, the adoption of GenAI in an enterprise setting requires additional security considerations to protect sensitive company data and maintain confidentiality. Enterprises should consider implementing some key security measures when leveraging generative AI solutions.
Restricting Training on Company Data: To ensure data privacy and prevent the exposure of proprietary or sensitive information, it is crucial to establish strict controls on the training process of generative AI models. Enterprises should avoid training their AI models directly on company-specific data. Instead, they can utilize pre-trained models or synthetic datasets that capture the desired characteristics without revealing confidential information. This approach minimizes the risk of unintentionally leaking proprietary knowledge or sensitive data through the generative AI training process.
Securing Generated Results: Enterprises must prioritize the security and privacy of the outputs generated by generative AI models. This involves implementing robust measures to control access to the generated results and prevent unauthorized dissemination. Encryption and access controls should be applied to ensure that only authorized personnel can access and share the generated content. By implementing strong encryption protocols and carefully managing access privileges, enterprises can safeguard valuable intellectual property and maintain control over the distribution and usage of the generated outputs.
Data Governance and Compliance: Enterprises need to establish comprehensive data governance frameworks and comply with relevant regulatory requirements. This includes defining policies and procedures for the collection, usage, retention, and disposal of data associated with generative AI systems. Data anonymization and de-identification techniques should be applied to protect the privacy of individuals and comply with data protection regulations. Regular audits and assessments should be conducted to ensure ongoing compliance and adherence to established security protocols.
Enhanced Authentication and Authorization: Enterprises should employ advanced authentication and authorization mechanisms to secure access to generative AI systems and data. Multi-factor authentication, strong password policies, and role-based access controls should be implemented to prevent unauthorized access. Additionally, user activity logging and monitoring can provide valuable insights into potential security breaches or suspicious activities, enabling timely intervention and response.
By implementing these enhanced security measures, enterprises can confidently leverage generative AI while minimizing the risks associated with sensitive company data and ensuring the privacy and confidentiality of generated results. By striking the right balance between innovation and security, businesses can unlock the vast potential of generative AI to drive growth and competitiveness while safeguarding their most valuable assets.
AI Provider Approaches to Addressing Security and Privacy Concerns
Generative AI has immense potential to revolutionize various industries. However, the proliferation of this technology has also raised valid concerns regarding security and data privacy. Companies like Google, OpenAI, and Meta AI recognize the importance of addressing these concerns and have implemented measures to mitigate risks. From stringent access controls and anonymization techniques to transparency and user consent, these companies prioritize privacy and data protection. While their approaches may differ slightly, all three companies actively engage in enhancing security measures, collaborating with the research community, and adhering to data protection regulations.
As Generative AI continues to advance, it is crucial for companies, researchers, and policymakers to collaborate closely to ensure the responsible and secure development and deployment of this technology. By prioritizing security and data privacy, we can unlock the full potential of generative AI while safeguarding the rights and interests of individuals and society as a whole.
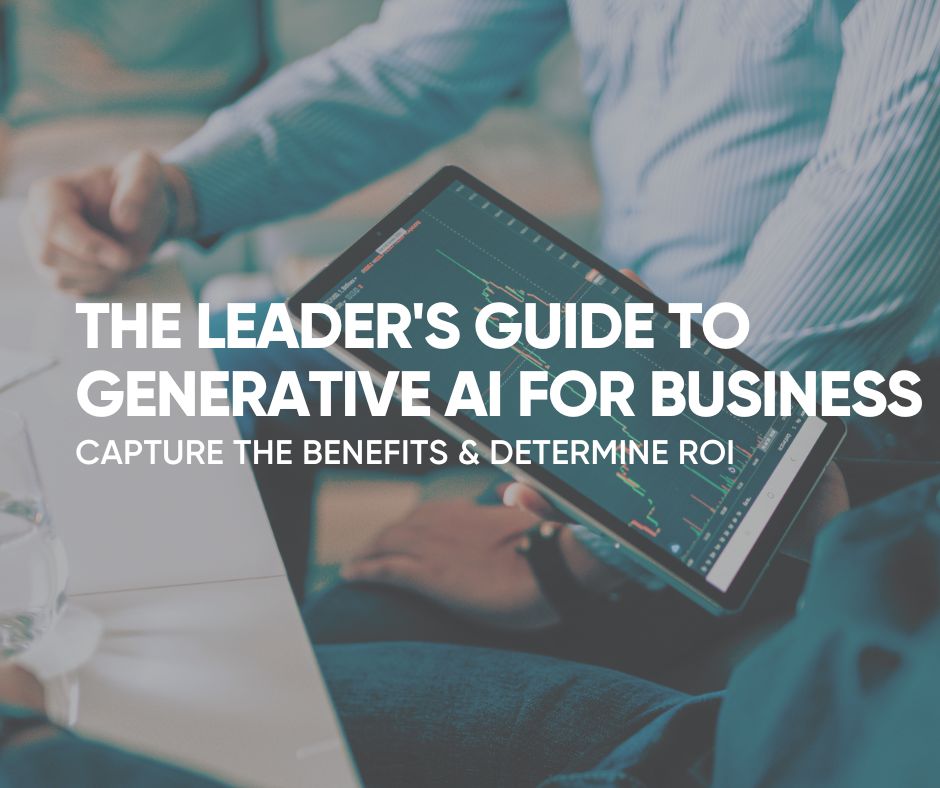
GENERATIVE AI EBOOK
The Leader’s Guide to Generative AI in Business
In “The Leader’s Guide” you will learn about the basics of generative AI, including how it works, the different types of generative AI models, and the benefits for enterprise organizations.
You will also learn about the challenges and opportunities associated with generative AI, and how you can elevate your business with this powerful new technology.